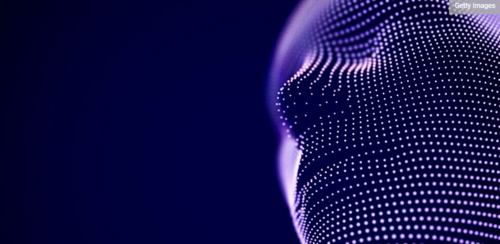
A Collision Course: AI Marketing, People and Process
In many cases, AI marketing continues to obscure reality.
What is artificial intelligence? Is a smart speaker truly intelligent? And what makes a factory, building, or even a home for that matter smart?
As for the first question, there are three key pieces, said Marcia Walker, principal consultant at SAS. “AI can learn from experience, adjust to new inputs, and accomplish tasks without manual intervention,” Walker said.
The term AI itself dates back to at least 1955, when four researchers, two hailing from academia and two from industry, proposed a research project to investigate the thesis that “every aspect of learning or any other feature of intelligence can in principle be so precisely described that a machine can be made to simulate it.”
If people must be present to continually fine-tune a program’s algorithm, it, therefore, cannot be said to possess artificial intelligence in the classical sense of the term. Yet AI marketing often uses the phrase in ways that are more artificial than intelligent. “Many times, I think the term ‘artificial intelligence’ is aspirational,” Walker said.
Smart in Name Only?
Is a smart speaker, which has become the prototypical smart home device, an example of artificial intelligence? Alas, no. At least not in the case of Amazon Alexa, which, according to Bloomberg, relies on a global team of thousands of people to listen to voice commands and help them fine-tune the assistant’s responses to specific queries in the future.
While Amazon describes Alexa as living “in the cloud” and “always getting smarter,” the voice assistant’s improved accuracy in understanding voice commands is at least partly a byproduct of manual intervention over time.
While the specific details about Alexa’s inner workings were new, that hasn’t stopped users from lamenting in recent years that the “smart speaker” didn’t live up to its name. “Amazon’s Alexa isn’t the future of AI — it’s a glorified radio clock,” wrote Quartz writer Alexander Aciman in 2017.
Gartner observed in its AI Hype Cycle report last year that the majority of conversational user interfaces, of which smart speakers are an example, remain “primitive, and thus are not able to respond to complex queries.”
Conversational user interfaces such as smart speakers also represent a continuing blurring of the lines between the Internet of Things, which is a broad technology trend that has received its own share of hype, and artificial intelligence, a word often applied undeservedly.
Of course, voice assistants, including Alexa, have improved steadily over the years. The venture capital firm Loup Ventures asked prominent smart speakers hundreds of queries and found, in December 2018, that Alexa correctly answered 72.5% of the time, its accuracy improved 9% compared to 12 months prior. By comparison, Google Home had an accuracy rate of 87.9% in December 2018.
While a firm scientific definition of intelligence, or even consciousness or thought, may be elusive, smart speakers won’t be passing the Turing test any time soon. And while the smart speaker is but one example, it is a convenient microcosm reflecting the gap between AI marketing and reality.
Looking for the “Invisible Hand of God”
While smart speakers may be an example of a technology that trails our expectations for intelligence, there is also a fear AI will have significant negative effects on at least some of humanity. While AI’s threat to automate jobs is likely the greatest social fear, Hollywood and a handful of public figures helped make the threat of an AI takeover famous, theorizing that advanced artificial intelligence poses an existential threat to society. The fact of the matter is: researchers widely disagree on when and if AI can develop an intelligence level matching or superseding human intelligence at large.
The computer scientist and AI researcher Jaron Lanier takes something of the opposite viewpoint. “The machines don’t mean a thing. They are barely even there without us,” Lanier said in one interview. “In order to support the fantasy of some kind of pure AI or freestanding AI, we are telling the people [who] supply the absolutely necessary data, that they are not needed.”
While analytics is fundamental to artificial intelligence, Walker said “I would argue the real base is intelligence, period. If we are going to talk about AI, we have to decide what is real intelligence.”
Critics have long accused tech companies of misrepresenting systems reliant on human input as artificial intelligence, but obscuring that fact. Some chess fans alleged as much after the 1997 victory of IBM’s Deep Blue chess engine over champion Garry Kasparov. Grandmasters Miguel Illescas, John Fedorowicz and Nick de Firmian helped provide Deep Blue with a database of openings and thousands of board configurations and hundreds of thousands of grandmaster games.
Kasparov won the first game in 45 moves. Before the second game, IBM enlisted an additional grandmaster, Joel Benjamin, to help hone the chess engine, which was allowed under the rules. Kasparov lost the next round, even though the engine, at one point, made an apparent blunder. Kasparov accused IBM of manually intervening during the game, which the rules forbade.
Referring to the famous human-machine matchup in a 2014 NPR interview, chess author Mig Greengard sympathized with Kasparov’s 1997 suspicions: “How could something play like God, then play like an idiot in the same game?” After the loss in the second game, Kasparov requested a summary of moves from Deep Blue’s recent games. He was denied access, although the Deep Blue team had access to hundreds of Kasparov’s games.
Deep Blue ultimately triumphed over Kasparov in game six of the match. While initially accusing IBM of cheating, he eventually accepted the chess engine’s superiority. “Deep Blue was intelligent the way your programmable alarm clock is intelligent. Not that losing to a $10 million alarm clock made me feel any better,” The Financial Times quotes him as saying. As for Deep Blue’s unwise move in the second game, IBM research scientist Murray Campbell explained it was a random glitch.
For IBM, Deep Blue was arguably its clearest AI marketing win until its Watson computer won Jeopardy in 2011. A number of chess buffs, however, continue to question Deep Blue’s victory, questioning the extent of human involvement in achieving that milestone. “They wonder if there was a sort of an ‘Invisible Hand of God,’ so to speak,” said Zulfikar Ramzan, chief technology officer or RSA, comparing the matchup to the controversial ‘Hand of God’ goal in the 1986 FIFA World Cup when Argentina defeated England. In that goal, the ball bounced off of Diego Maradona’s hand beforehand. A referee said he didn’t see the infringement.
The 1997 chess match between Kasparov and Deep Blue could have established a scientific precedent for artificial intelligence, but it didn’t. “We lost the opportunity to understand whether there was something novel about how chess was being played and whether you could apply these types of [computing] techniques to problems previously thought you couldn’t apply them to,” Ramzan said. “For a long time, people thought chess required human intuition.” But the collaboration between grandmasters and computer scientists over the years has resulted in even free chess engines such as Stockfish with scores considerably higher than those of the top-ranked human players of all time.
A game engine from Google known as AlphaGo also defeated a human in 1997, which was another game where at least some experts felt humans had the upper hand. “It may be a hundred years before a computer beats humans at Go — maybe even longer,” Piet Hut, Ph.D. an astrophysicist at the Institute for Advanced Study told The New York Times in 1997. “If a reasonably intelligent person learned to play Go, in a few months he could beat all existing computer programs. You don’t have to be a Kasparov.”
Enough With the Games
But deploying artificial intelligence or related techniques such as machine learning, deep learning, and analytics to thorny real-world problems — for instance, predicting when complex machines will fail or how to make health care or a cluster of factories more efficient — can still be challenging. “Oftentimes, it’s not just a matter of deploying a technique to help solve a problem, but understanding the domain and all of the context around it,” Ramzan said. Hidden biases can also creep into algorithms, or they could be sabotaged by ill-willed humans.
The clear victories of AI-themed demonstrations such as Deep Blue’s victory in chess, AlphaGo’s victory in Go, and broader applications of machine learning to solve concrete specified problems helped fuel the imaginations of marketers promising their software can revolutionize any company’s business. Attending a trade show dedicated to the industrial sector highlights the sheer number of companies promising to help any company revolutionize your business with AI and other cutting edge technologies. “There’s a flurry of buzzwords that don’t mean anything,” said Saar Yoskovitz, co-founder and CEO of Augury, which uses a combination of hardware and algorithms to monitor the health of industrial machinery.
While the promise of implementing techniques like machine learning to drive transformation within industrial environments are well-founded, Yoskovitz laments many general industrial techniques used in the sector are old-fashioned. “The tools haven’t changed since the 1980s in some cases,” Yoskovitz said. “There are flip charts, and maybe an earpiece system where you can log maintenance.”
Part of the challenge is that while leading industrial companies have experience in analytics, machine learning, and so forth, those techniques haven’t historically been a core focus. “They tend to be really good at making equipment to run big industrial processes, but that’s a different skill set than understanding big data and what it takes to do true advanced analytics,” Walker said. “It’s a parallel world.” In general, industrial companies pursuing projects under the artificial intelligence umbrella may struggle with basics such as preparing data for analytics.
A gradual change is underway, however, toward software-as-a-service applications that could potentially change how industrial environments are managed. “It should — I say should because it is not yet — be truly revolutionary,” Yoskovitz added. But in order to drive such a transformation, and to unleash the potential of AI, ML, and related techniques, requires changes to people and process. “I’ll give you one example of this from the software world. Look at what happened to sales organizations in the past decade,” Yoskovitz said. “It used to be that people looked at quarterly revenues. And today, you would call that a lagging indicator, not a leading indicator.” SaaS for sales staff made it simpler to track how many phone calls sales reps make along with their conversion rates. Sales professionals can use that information to “tweak their messaging because whatever change we make today will affect revenue in six months,” Yoskovitz said. “So how do we, very similarly, in our industry go from lagging indicators — uptime, for instance, is a lagging indicator — to leading indicators?”
Organizations that can come up with firm answers to such questions, matched with a careful application of technologies will find themselves ahead of the vast majority of the competition. Doing so requires aligning the three elements of the people, process, and technology framework. Walker concluded: “And I think, for us to be really successful at AI, we also have to be really successful at looking at what it means to be human.”
Written by Brian Buntz of IOT World Today
https://www.iotworldtoday.com/2019/05/02/a-collision-course-ai-marketing-people-and-process/
TP